I recently worked on some Python code to detect which are the main colors in an image.
To do that, my images were stored in an Oracle Cloud Infrastructure block storage bucket.
The process had to be done in 3 steps:
- I had first to extract them by using the “oci” python package.
- Then I had to convert the unstructured binary image to a structured numpy array.
- And finally, I used an unsupervised ML routine (KMeans Clustering) to analyze the numpy array and detect which were the main colors in this image.
Reading Images stored in an OCI block storage bucket
To read images, or more generally, files store in an OCI block storage bucket. You need to have configured your client environment to access the OCI.
To do that, you will need various OCIDs (user, tenant), some keys (private and public). I will not develop this part because I already did it in a previous post … see here !
Once your configuration is ok, you have to load it into your python script, get an ObjectStorageClient object from the configuration, and request the namespace data of your ObjectStorageClient.
After that, it becomes easy to read an object (file) inside a bucket referenced inside the namespace.
This is done by the following code
compartment_id = config["tenancy"]
object_storage = oci.object_storage.ObjectStorageClient(config)
namespace = object_storage.get_namespace().data
bucket_name="python-bucket"
object_name="union_jack.jpg"
my_object = object_storage.get_object(namespace,
bucket_name,
object_name)
print("type(my_object.data.content) = ",type(my_object.data.content))
As you can see, I printed the class type of the object content … and without any surprise, it’s a “bytes” class.
type(my_object.data.content) = <class 'bytes'>
Note: If your images are stored by another cloud provider. They usually have a Python SDK in order to do the same things 😉
Converting an unstructured binary image to a numpy array
Once I did that, if I want to process my image I have to convert it in a usable data structure. And, with Python, the best data structure to process images is a numpy array, so I had to find a way to convert my binary soap (Bytes) to a structures numpy array.
As I don’t want to use a temporary file to do that stuff, I used a BytesIO object to process them directly in memory. At the end of the stream, I used a pillow Image (new name for the deprecated PIL package) from the BytesIO stream.
After that, a conversion to a numy array was possible. Please note that I had to convert a bit my numpy array structure. As you may know, an image file is represented in a multi-dimension array.
The first two dimensions represent the pixels of your Image. Added to that, you have 3rd dimension which encode for Red, Green and Blue values of each pixel. Sometimes a fourth value is added for what is called “Alpha” which is intended in transparency encoding. As I don’t know how were encoded Images, and as I don’t need to process the Alpha layer, I converted my 3 or 4 layers array into a 3 layers array (R,G and B encoding only).
The following code do the stuff:
from PIL import Image
from io import BytesIO
im=Image.open(BytesIO(my_object.data.content))
img=np.array(im)[:,:,:3]
print("img.shape=",img.shape)
This will produce the result below:
img.shape= (640, 1280, 3)
So my image is represented by a numpy array (ndarray). my image width is 640 pixels, height is 1280 pixels and each pixel is encoded by 3 values for Red, Green and Blue.
Using a clustering ML algorithm to detect colors
Next step, but not least. We have to choose a method to detect colors in the image.
First, I thought about getting the “average” color, but doing this is not a good way, because in the case of your image is equally colored by yellow, blue, red, and green … your average color will be a crappy brown which is not realistic.
The best way to get colors is to run a unsupervised machine learning algorithm (K-Means) to group all your colors into clusters based on R, G and B values. No matter the ML framework you will use to execute the KMeans, after execute your program you will get, the center point of each cluster which represent the color associated with the cluster and the differents labels for your clusters. Then you will be able to count the number of occurence of your label, and you will get the number of points inside your cluster.
It becomes easy to count the number of points in each color, this is for the most important thing in this algorithm. The other key point is how to structure your data as input for your KMeans.
This is simply resolved by flattening your image representation (in the numpy array). The array is flatten to a one-dimension list of triplets (reprensenting your RGB values).
In the following code, I used opencv (cv2 package) which is often used for image detection and capturing. This package is delivered with a kmeans algorithm that is optimized for image processing.
import cv2
# pixels is the 1D array, results of the img flattening process (made by reshape function)
pixels = np.float32(img.reshape(-1, 3))
print("Pixel shape = ", pixels.shape)
# Here is the number of colors we are trying to detect.
n_colors = 5
# Opencv kmeans parameters (See the following URL for more information:
# https://docs.opencv.org/3.0-beta/doc/py_tutorials/py_ml/py_kmeans/py_kmeans_opencv/py_kmeans_opencv.html
criteria = (cv2.TERM_CRITERIA_EPS + cv2.TERM_CRITERIA_MAX_ITER, 200, .1)
flags = cv2.KMEANS_RANDOM_CENTERS
# palette represents clusters centers
# Labels represents the cluster labels.
# As we have 5 colors, labels are 0,1,2,3,4
_, labels, palette = cv2.kmeans(pixels, n_colors, None, criteria, 10, flags)
# And counts represents the number of occurence for each label
_, counts = np.unique(labels, return_counts=True)
# Our dominant color is the color that have the maximum number of occurence in the "counts" array
dominant = palette[np.argmax(counts)]
print("dominant color (RVB) =",dominant)
If you prefer to use tensorflow, the code below will do the stuff
import tensorflow as tf
# this is for removing all the tensorflow INFO and WARN messages
tf.logging.set_verbosity(tf.logging.ERROR)
# pixels is the 1D array, results of the img flattening process (made by reshape function)
pixels = np.float32(img.reshape(-1, 3))
print("Pixel shape = ", pixels.shape)
def input_fn():
return tf.train.limit_epochs(tf.convert_to_tensor(pixels, dtype=tf.float32), num_epochs=1)
n_colors = 5
kmeans = tf.contrib.factorization.KMeansClustering(num_clusters=n_colors,
use_mini_batch=False)
num_iterations = 20
for _ in range(num_iterations):
kmeans.train(input_fn)
print('Training ... score:', kmeans.score(input_fn))
cluster_centers = kmeans.cluster_centers()
cluster_indices = list(kmeans.predict_cluster_index(input_fn))
counts=np.unique(cluster_indices, return_counts=True)[1]
palette=cluster_centers
dominant = palette[np.argmax(counts)]
print("dominant =",dominant)
Now we have our results, we are able to produce a nice plot with:
- the initial picture,
- the dominant colors gradient,
- the main dominant color
- the second dominant color (I did that because In the code I worked on, many pictures had a white background which was detected and the main color in 99% of the cases)
And to do that, I used the matplotlib library:
import matplotlib as mpl
%matplotlib notebook
from matplotlib import pyplot as plt
indices = np.argsort(counts)[::-1]
freqs = np.cumsum(np.hstack([[0], counts[indices]/counts.sum()]))
rows = np.int_(img.shape[0]*freqs)
dom_patch = np.zeros(shape=img.shape, dtype=np.uint8)
main_patch=np.ones(shape=img.shape, dtype=np.uint8)*np.uint8(palette[indices[0]])
second_patch=np.ones(shape=img.shape, dtype=np.uint8)*np.uint8(palette[indices[1]])
for i in range(len(rows) - 1):
dom_patch[rows[i]:rows[i + 1], :, :] += np.uint8(palette[indices[i]])
fig, (ax0, ax1, ax2, ax3 ) = plt.subplots(1, 4 , figsize=(9,6))
ax0.imshow(img)
ax0.set_title('Original')
ax0.axis('off')
ax1.imshow(dom_patch)
ax1.set_title('Dominant colors')
ax1.yaxis.set_major_locator(plt.NullLocator())
ax1.xaxis.set_major_locator(plt.NullLocator())
ax2.imshow(main_patch)
ax2.set_title('Main color')
ax2.yaxis.set_major_locator(plt.NullLocator())
ax2.xaxis.set_major_locator(plt.NullLocator())
ax3.imshow(second_patch)
ax3.set_title('Second color')
ax3.yaxis.set_major_locator(plt.NullLocator())
ax3.xaxis.set_major_locator(plt.NullLocator())
plt.show(fig)
Please note that, this code was running inside a jupyter notebook … so adapt the code if you want to run it in another context.
This will produce that kind of result :
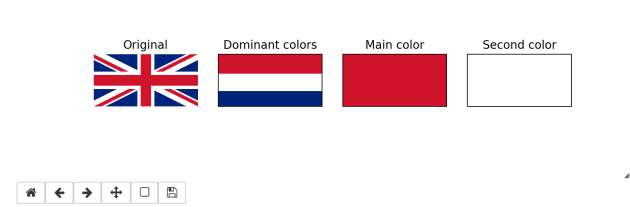
